New algorithm takes both complexity and risk out of written procedures
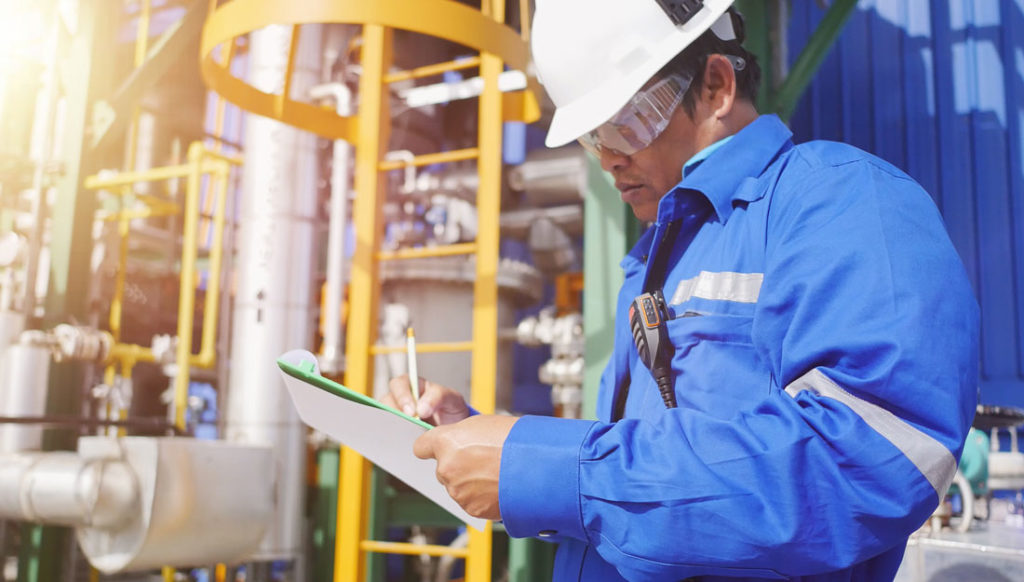
Correctly executing tasks can often be a matter of life and death in high-risk industrial settings like nuclear power plants and petrochemical facilities. Written procedures are a common way to ensure operating tasks are performed correctly; however, 49 of the 100 incidents in oil and gas industries over the past two decades included some sort of procedural error. One explanation for procedural errors is procedures that are ambiguous, overly complex or that require judgment or decision making from operators. Having a reliable way to evaluate written procedures could lead to clearer and more easily followed procedures.
A study published in the International Journal of Industrial Ergonomics discusses an aspect of written procedures called step-level complexity and proposes a software-based evaluation method that measures whether a written procedure has problems that could make them more difficult to follow.
In this study, S. Camille Peres, associate professor at the Texas A&M University School of Public Health, along with Farzan Sasangohar, Nilesh Ade, Noor Quddus, and Pranav Kannan, of the Texas A&M College of Engineering, used existing frameworks for evaluating procedures to build an algorithm to test written procedures.
Prior research has led to methods for evaluating task complexity, but these methods have not been practical. They often need information outside of the procedures themselves, such as task goals, and make heavy use of limited time and resources. The novel method Peres and colleagues have developed attempts to avoid these limitations, with the goal of both identifying overly complex procedures in need of revision and guiding procedure writers when creating new procedures.
The researchers focused on four types of tasks and five task characteristics when developing their evaluation method. They set up a natural language processing (NLP) machine learning algorithm trained with a vocabulary of 400,000 words. Peres and colleagues used the NLP model to determine which steps required operator judgment or decisions, contained multiple sub-steps or were larger in size or contained large amounts of information. They then tested the model using data from upstream and downstream oil and gas facilities.
The NLP algorithm identified the various sources of task complexity with accuracies ranging from 60 to 79.3%. By identifying potentially complex steps, procedure writers can either revise existing steps or create new steps with lower levels of complexity.