Software can sort illicit materials from mixed nuclear samples, study says
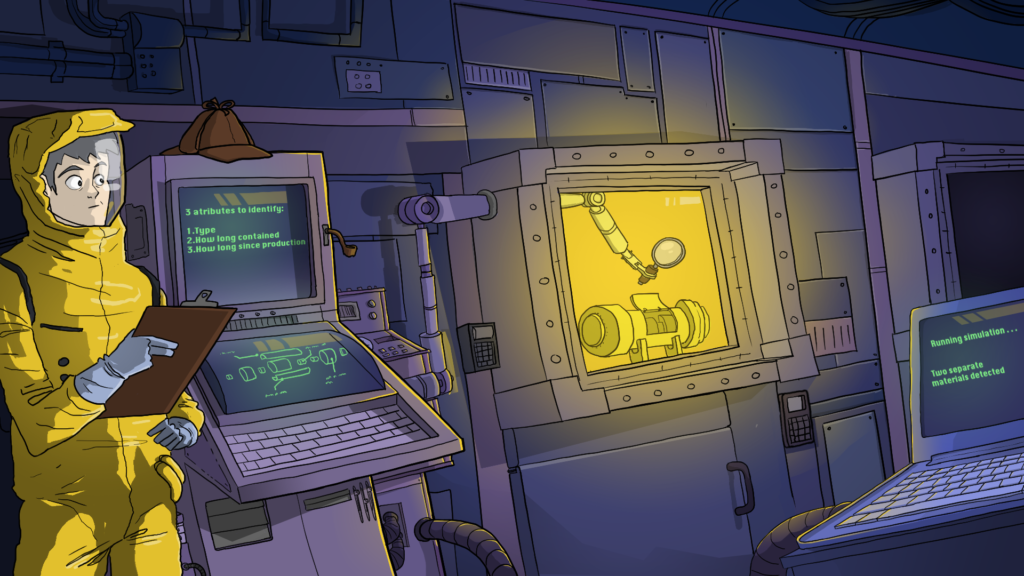
Millions of shipments of nuclear and other radiological materials are moved in the United States every year for good reasons, including health care, power generation, research and manufacturing. But there remains the threat that bad actors in possession of stolen or illegally produced nuclear materials or weapons will try to smuggle them across borders for nefarious purposes.
Texas A&M University researchers are making it harder for them to succeed.
If border agents intercept illicit nuclear materials, investigators need to know who produced them and where they came from. Fortunately, nuclear materials carry certain forensic markers that can reveal valuable information, much like fingerprints can identify criminals.
For instance, when scientists examine the concentration of certain key contaminant isotopes in separated plutonium samples they can determine three different attributes of the sample’s history: the type of nuclear reactor that produced it, how long the plutonium or uranium was contained in the reactor and how long ago it was produced.
With current statistical methodologies, they can determine these three attributes utilizing a generated database that stores the required information as a mathematical variation of these attributes for various nuclear reactor types and emerge with a good idea of who made the material.
“But what if investigators are presented with a mixed plutonium sample?” said Sunil Chirayath, author of a new study on nuclear forensics recently published in the journal Nuclear Science and Engineering. “Suppose the adversary is mixing materials from two nuclear reactors at two different times, and that material is cooled for different times. A bad actor might do this intentionally to disguise it.”
Mixed samples of nuclear material are significantly more challenging to identify with traditional methodologies. In a real-world situation, the extra time required could have a catastrophic impact on the global community.
To improve the process, Chirayath, associate professor in the Department of Nuclear Engineering and director of the Texas A&M Engineering Experiment Station’s Center for Nuclear Security Science and Policy Initiatives, along with his research team, has developed a methodology using machine learning, a type of artificial intelligence.
He can produce identifying markers through simulations, and then store that data in a 3D database. Each attribute is one level of the database, and a standard computer can quickly process the data and lead investigators to the reactor type that produced the plutonium sample — and, potentially, the suspects — by joining other pieces of the puzzle gathered through traditional forensics.
Three experiments of irradiating uranium using three different reactor types and post-irradiation examinations have been conducted at Texas A&M to date. Without knowing the samples’ origins, doctoral student researcher Patrick O’Neal successfully identified where each of the plutonium samples was produced by using machine learning.