Deep-learning model predicts mRNA degradation to improve stability in thermally sensitive vaccines
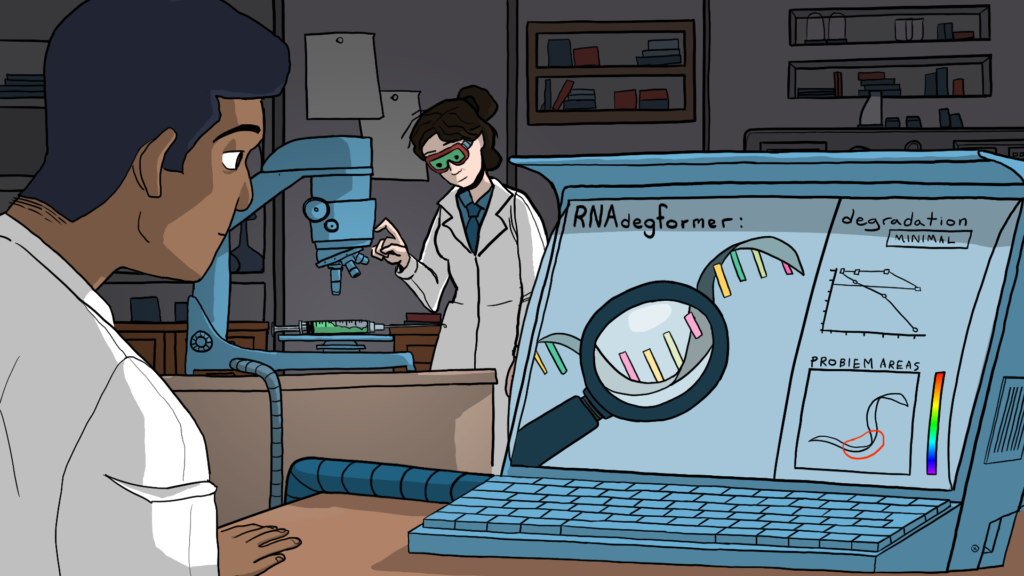
Ryan Farrell/Division of Research
Messenger Ribonucleic Acid (mRNA) as a therapeutic approach is gaining momentum due to its ability to be rapidly manufactured and its promising outcomes.
However, mRNA-based therapeutics can face challenges due to their thermal instability, which makes them susceptible to chemical degradation. As a result, mRNA vaccines require stringent conditions for manufacturing, storage and worldwide delivery. To make mRNA vaccines more broadly accessible, it is critical to understand and improve their stability.
Dr. Qing Sun, professor in the Artie McFerrin Department of Chemical Engineering at Texas A&M University, and a team of graduate students have created an effective and interpretable model architecture using deep-learning techniques that can predict RNA degradation more accurately than previous best methods, such as Degscore models, RNA folding algorithms and other machine-learning models.
Their model was tested to show its efficiency, and the findings were recently published in Briefings in Bioinformatics.
“mRNA’s inherent thermal instability has hampered the distribution of mRNA vaccines worldwide due to in-line hydrolysis, a chemical degradation reaction,” said Sun. “For this reason, our research seeks to understand and predict mRNA degradations.”
To combat this problem, Sun and her team turned to deep-learning techniques, in which they developed the RNAdegformer — a deep-learning-based model powered by artificial neural networks capable of extracting data and using these insights to make predictions.
According to Sun, the RNAdegformer processes RNA sequences with self-attention and convolutions, two deep-learning techniques that have proved dominant in the fields of computer vision and natural language processing while utilizing the biophysical features of RNA secondary structure features and base pairing probabilities.
“The RNAdegformer outperforms previous best methods at predicting degradation properties at the nucleotide level, which are like letters of a sentence that combine to form mRNA,” said Sun. “We can make predictions about each nucleotide in COVID-19 mRNA vaccines. RNAdegformer predictions also exhibit improved correlation with RNA in vitro half-life compared with previous best methods.”